Quadratic Regression
Quadratic regression is a statistical method used to model a relationship between variables with a parabolic best-fit curve, rather than a straight line. It's ideal when the data relationship appears curvilinear. The goal is to fit a quadratic equation to the observed data, providing a nuanced model of the relationship. Contrary to historical or biological connotations, "regression" in this mathematical context refers to advancing our understanding of complex relationships among variables, particularly when data follows a curvilinear pattern.
What is quadratic regression?
We have already covered linear regression, which we use to predict the value of a variable based on the value of another variable. This gives us the "line of best fit" and allows us to make accurate predictions about our data.
The same general concept applies to quadratic regression. The only difference is that instead of trying to find the line of best fit, we're trying to find the parabola of best fit.
So how exactly does this work?
Instead of getting a simple linear equation, quadratic regression leaves us with this:

If you already know about the "least squares method," you can use the exact same process with quadratic regression.
We need to find the values of , , and so that the squared vertical distance between each point and the quadratic curve is minimal.
We can use a matrix equation to represent our quadratic curve:
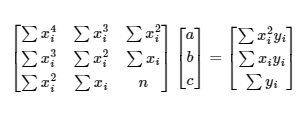
We can also use these formulas to find the values of , , and :
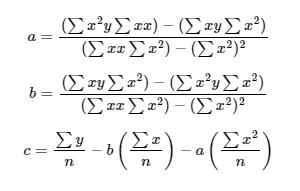
In these equations:
- and are our variables
- , , and are our coefficients for our quadratic equation
- the number of elements
- the sum of values
- = the sum of values
- the sum of the squares of values
- the sum of the cubes of values
- the sum of the fourth powers of the values
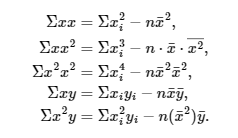
These calculations can be represented by a system of equations in a matrix form, and the coefficients a, b, and c can be determined using these equations. It's important to note that the equations and methods used to calculate these coefficients can be complex, involving several sums and squares of various combinations of the data points. Thus, these computations are typically done with the assistance of software.
Note that the relative predictive power of a quadratic model is denoted by R2. Our predictive power tells us how accurate our predictions truly are. It is the power of a scientific theory to generate testable predictions. In the case of quadratic regression, this applies to the predictions related to our parabola.
The formula for our predictive power is as follows:
In this formula:
Our relative predictive power lies anywhere between 0 and 1. The closer it is to 1, the more accurate our model is.
We should also note that the "bar" above the y value in the SST equation represents the average of all y values.
Working with quadratic regression
As we might have guessed, these calculations can become quite complex and tedious. We have just gone over a few very detailed formulas, but the truth is that we can handle these calculations with a graphing calculator. This saves us from having to go through so many steps -- but we still must understand the core concepts at play.
Let's try a practice problem that includes quadratic regression. Consider the following set of data:
Can we determine the quadratic regression for this set?
Our first step is to enter our x-coordinates and y-coordinates into our graphing calculator. We can then carry out our operation for a quadratic equation. This will give us the equation of the parabola that best approximates the points:
Great! Now all we need to do is plot our graph. We should be left with something like this:
We also know that our relative predictive power ( ) is 0.9942. That's pretty accurate -- and it tells us that our calculations for quadratic regression worked!
Topics related to the Quadratic Regression
Developing a Probability Distribution from Empirical Data
Line of best fit(Eyeball Method)
Flashcards covering the Quadratic Regression
Common Core: High School - Statistics and Probability Flashcards
Practice tests covering the Quadratic Regression
Probability Theory Practice Tests
Common Core: High School - Statistics and Probability Diagnostic Tests
Pair your student with a tutor who understands quadratic regression
Quadratic regression is similar to linear regression, but there are a few distinctions that can catch students off guard. The best way to address these potential points of confusion is alongside a qualified, experienced math tutor in a 1-on-1 learning environment. Tutors can slow things down to a more manageable pace for your student, allowing them to walk through the concepts step-by-step. They can also break down more complicated steps into manageable chunks. In addition, a tutor can give your student plenty of opportunities to ask questions -- something that isn't always possible in a full classroom. While tutoring can make things more manageable, it can also present interesting challenges -- and your student can take the time to practice their skills. Speak with our Educational Directors to learn more, and rest assured: Varsity Tutors will pair your student with a suitable tutor.
- ACT Test Prep
- Rhode Island Bar Exam Test Prep
- BCABA - Board Certified Assistant Behavior Analyst Test Prep
- Actuarial Exam FM Courses & Classes
- CogAT Courses & Classes
- AWS Certified Cloud Practitioner Test Prep
- SolidWorks Training
- CPFA Courses & Classes
- CSET - California Subject Examinations for Teachers Test Prep
- Middle School Writing Tutors
- Series 57 Courses & Classes
- Missouri Bar Exam Test Prep
- FTCE - Florida Teacher Certification Examinations Test Prep
- Series 65 Test Prep
- Minnesota Bar Exam Test Prep
- AFOQT - Air Force Officer Qualifying Test Test Prep
- CGEIT - Certified in the Governance of Enterprise IT Courses & Classes
- Liberal Arts Math Tutors
- Exam SRM - Statistics for Risk Modeling Test Prep
- Destiny 2 Tutors