All Common Core: High School - Statistics and Probability Resources
Example Questions
Example Question #1 : Develop Probability Distribution For Random Variable With Empirically Assigned Probabilities: Ccss.Math.Content.Hss Md.A.4
A statistics class has ten homework assignments. The first two assignments take 2 hours each to complete. The next three assignments take 4 hours each to complete. The three after that take 10 hours each, and the final two assignments take 15 hours each. On average, how long does it take to complete a homework assignment?
15.5 hours
7.6 hours
12.6 hours
9.8 hours
5 hours
7.6 hours
Example Question #2 : Develop Probability Distribution For Random Variable With Empirically Assigned Probabilities: Ccss.Math.Content.Hss Md.A.4
In a particular African coastal region, seismologists studied tsunamis by observing seismic shifts and earthquakes in the South Pacific Ocean. During their study, they were able to note the number of earthquakes as well as the number of resulting tsunamis in this region. They observed earthquakes and found that there was a
probability of a tsunami hitting the region in the next year based on previous data. What is the probability that the region will have
tsunamis in the next year?
Cannot be determined
This standard requires us to work backwards in order to deconstruct and solve a problem. At their core, questions in this standard ask us to make approximations about sample statistics while using population parameters and distributions. In other words, these standards use real data to help us approximate values of sample characteristics. It is important to note that these questions—in particular—utilize real data. This means that the data is collected in studies through the observation of real-world situations rather than examples of probabilities calculated through theoretical scenarios.
In these questions, students will be asked to use selections of sample data to calculate probabilities be able to identify expected values; thus, we need to be familiar with how to determine what the problem is asking you to calculate (i.e. probability, expected value, or actual average) and how to apply the lessons learned in the expected means and probability distribution model lessons. First, we will discuss probabilities in a general sense.
A probability is generally defined as the chances or likelihood of an event occurring. It is calculated by identifying two components: the event and the sample space. The event is defined as the favorable outcome or success that we wish to observe. On the other hand, the sample space is defined as the set of all possible outcomes for the event. Mathematically we calculate probabilities by dividing the event by the sample space:
Let's use a simple example: the rolling of a die. We want to know the probability of rolling a one. We know that the sample space is six because there are six sides or outcomes to the die. Also, we know that there is only a single side with a value of one; therefore,
Now, let's convert this into a percentage:
Probabilities expressed in fraction form will have values between zero and one. One indicates that an event will definitely occur, while zero indicates that an event will not occur. Likewise, probabilities expressed as percentages possess values between zero and one hundred percent where probabilities closer to zero are unlikely to occur and those close to one hundred percent are more likely to occur.
According to this logic, we would expect to roll a particular number on a die one out of every six rolls; however, we may roll the same number multiple times or not at all in six rolls. This discrepancy creates a difference between the expected mean and the actual mean. The expected mean is a hypothetical calculation that assumes a very large sample size and no intervening variables (such as differing forces on the roll and changes in the friction of the surface the die is rolled upon between rolls). Under these conditions we can calculate that any number on the die in a "perfect world" should be one out of six. On the other hand the true or actual mean is calculated using "real" date. In these calculations we would roll a die a particular number of times and use it to develop a probability of rolling a particular number. It is important to note that, theoretically, actual means will eventually equal expected means over a large—or near infinite—amount of trials. In other words, over many many rolls we would eventually find that each number on the die has a one in six chance of being rolled.
Now, let's discuss how expected means are determined. The expected mean is calculated using the following formula:
In this equation the variables are identified as the following:
We can illustrate this using an example problem. A list of individuals and the respective size of their motorcycles in cubic inches is provided. If you were to randomly select any one person from the list, then what size would you expect their motorcycle to be?
Let's use this information to solve the problem. In order to solve the motorcycle problem, we need to use the expected mean formula. We will substitute each of the motorcycle owner's engine size with its respective probability and solve.
Each of the values have the same probability of being chosen—one out of eleven. As a result, we can simplify this equation:
Round to the nearest one's place.
Note that we can solve this problem in this simplified manner because all of the people had an equal probability of being chosen. If his or her probabilities differed, then we would need to substitute in each person’s respective probability of being chosen.
Finally, we need to determine how we will discuss how to create a probability distribution graph for a probability model. We will use the following equation to calculate the probabilities to be used in this graphical display:
Remember that in combinations and permutations a combination is calculated using the following formula:
Now, we can write the following formula.
In this formula variables are defined in the following manner:
Let's investigate this standard through the use of an example. Suppose a researcher rolls a die twelve times and notes whether the die rolls on an even or an odd number. If the die is fair (i.e. every number has an equal probability of being rolled or each roll is random), then would the probability distribution graph follow the pattern of a normal distribution? Lets create a table and solve for each variable. The probability of rolling an even number—a two, four, or a six— is three out of six or fifty percent. Likewise, the probability of failure (i.e. rolling an odd number) is three out of six or fifty percent. Next, we need to list the number of successes for each event—variable . The researcher can roll an even number every time he rolls and he may not even roll an even number in all twelve trial. We need to calculate this probability for every possible number of successful events; therefore, the number of successes ranges from zero to twelve. Last, we know that there are a total of twelve trials. We have solved for the probability of each of these variables for every possible number of successes in the trials.
Once this data is tabulated we can graph the probability of rolling an even number. If we look at the graph then we can see if it follows the bell shape curve of a normal distribution.
A bell curve is shaped like the following image:
We can quickly tell that the graph of the probability distribution does follow the shape of a normal or "bell" curve. Now we can use this information to solve the given question.
In the question we are told that the seismologists observed earthquakes. We also know that the probability of an earthquake creating a tsunami is
. Now, we need to find out the probability of three tsunamis hitting the region in the next year. In order to do so, we will use the following equation:
We need to solve for the following probability:
Now, we need to name our variables:
Now, we can substitute in these variables and solve our equation.
Simplify.
Solve and remember to follow the order of operations.
Reduce.
Example Question #3 : Develop Probability Distribution For Random Variable With Empirically Assigned Probabilities: Ccss.Math.Content.Hss Md.A.4
In a particular African coastal region, seismologists studied tsunamis by observing seismic shifts and earthquakes in the South Pacific Ocean. During their study, they were able to note the number of earthquakes as well as the number of resulting tsunamis in this region. They observed earthquakes and found that there was
probability of a tsunami hitting the region in the next year based on previous data. What is the probability that the region will have
tsunami in the next year?
Cannot be determined
This standard requires us to work backwards in order to deconstruct and solve a problem. At their core, questions in this standard ask us to make approximations about sample statistics while using population parameters and distributions. In other words, these standards use real data to help us approximate values of sample characteristics. It is important to note that these questions—in particular—utilize real data. This means that the data is collected in studies through the observation of real-world situations rather than examples of probabilities calculated through theoretical scenarios.
In these questions, students will be asked to use selections of sample data to calculate probabilities be able to identify expected values; thus, we need to be familiar with how to determine what the problem is asking you to calculate (i.e. probability, expected value, or actual average) and how to apply the lessons learned in the expected means and probability distribution model lessons. First, we will discuss probabilities in a general sense.
A probability is generally defined as the chances or likelihood of an event occurring. It is calculated by identifying two components: the event and the sample space. The event is defined as the favorable outcome or success that we wish to observe. On the other hand, the sample space is defined as the set of all possible outcomes for the event. Mathematically we calculate probabilities by dividing the event by the sample space:
Let's use a simple example: the rolling of a die. We want to know the probability of rolling a one. We know that the sample space is six because there are six sides or outcomes to the die. Also, we know that there is only a single side with a value of one; therefore,
Now, let's convert this into a percentage:
Probabilities expressed in fraction form will have values between zero and one. One indicates that an event will definitely occur, while zero indicates that an event will not occur. Likewise, probabilities expressed as percentages possess values between zero and one hundred percent where probabilities closer to zero are unlikely to occur and those close to one hundred percent are more likely to occur.
According to this logic, we would expect to roll a particular number on a die one out of every six rolls; however, we may roll the same number multiple times or not at all in six rolls. This discrepancy creates a difference between the expected mean and the actual mean. The expected mean is a hypothetical calculation that assumes a very large sample size and no intervening variables (such as differing forces on the roll and changes in the friction of the surface the die is rolled upon between rolls). Under these conditions we can calculate that any number on the die in a "perfect world" should be one out of six. On the other hand the true or actual mean is calculated using "real" date. In these calculations we would roll a die a particular number of times and use it to develop a probability of rolling a particular number. It is important to note that, theoretically, actual means will eventually equal expected means over a large—or near infinite—amount of trials. In other words, over many many rolls we would eventually find that each number on the die has a one in six chance of being rolled.
Now, let's discuss how expected means are determined. The expected mean is calculated using the following formula:
In this equation the variables are identified as the following:
We can illustrate this using an example problem. A list of individuals and the respective size of their motorcycles in cubic inches is provided. If you were to randomly select any one person from the list, then what size would you expect their motorcycle to be?
Let's use this information to solve the problem. In order to solve the motorcycle problem, we need to use the expected mean formula. We will substitute each of the motorcycle owner's engine size with its respective probability and solve.
Each of the values have the same probability of being chosen—one out of eleven. As a result, we can simplify this equation:
Round to the nearest one's place.
Note that we can solve this problem in this simplified manner because all of the people had an equal probability of being chosen. If his or her probabilities differed, then we would need to substitute in each person’s respective probability of being chosen.
Finally, we need to determine how we will discuss how to create a probability distribution graph for a probability model. We will use the following equation to calculate the probabilities to be used in this graphical display:
Remember that in combinations and permutations a combination is calculated using the following formula:
Now, we can write the following formula.
In this formula variables are defined in the following manner:
Let's investigate this standard through the use of an example. Suppose a researcher rolls a die twelve times and notes whether the die rolls on an even or an odd number. If the die is fair (i.e. every number has an equal probability of being rolled or each roll is random), then would the probability distribution graph follow the pattern of a normal distribution? Lets create a table and solve for each variable. The probability of rolling an even number—a two, four, or a six— is three out of six or fifty percent. Likewise, the probability of failure (i.e. rolling an odd number) is three out of six or fifty percent. Next, we need to list the number of successes for each event—variable . The researcher can roll an even number every time he rolls and he may not even roll an even number in all twelve trial. We need to calculate this probability for every possible number of successful events; therefore, the number of successes ranges from zero to twelve. Last, we know that there are a total of twelve trials. We have solved for the probability of each of these variables for every possible number of successes in the trials.
Once this data is tabulated we can graph the probability of rolling an even number. If we look at the graph then we can see if it follows the bell shape curve of a normal distribution.
A bell curve is shaped like the following image:
We can quickly tell that the graph of the probability distribution does follow the shape of a normal or "bell" curve. Now we can use this information to solve the given question.
In the question we are told that the seismologists observed earthquakes. We also know that the probability of an earthquake creating a tsunami is
. Now, we need to find out the probability of three tsunamis hitting the region in the next year. In order to do so, we will use the following equation:
We need to solve for the following probability:
Now, we need to name our variables:
Now, we can substitute in these variables and solve our equation.
Simplify.
Solve and remember to follow the order of operations.
Rewrite in scientific notation.
Example Question #371 : High School: Statistics & Probability
In a particular African coastal region, seismologists studied tsunamis by observing seismic shifts and earthquakes in the South Pacific Ocean. During their study, they were able to note the number of earthquakes as well as the number of resulting tsunamis in this region. They observed earthquakes and found that there was
probability of a tsunami hitting the region in the next year based on previous data. What is the probability that the region will have
tsunamis in the next year?
Cannot be determined
This standard requires us to work backwards in order to deconstruct and solve a problem. At their core, questions in this standard ask us to make approximations about sample statistics while using population parameters and distributions. In other words, these standards use real data to help us approximate values of sample characteristics. It is important to note that these questions—in particular—utilize real data. This means that the data is collected in studies through the observation of real-world situations rather than examples of probabilities calculated through theoretical scenarios.
In these questions, students will be asked to use selections of sample data to calculate probabilities be able to identify expected values; thus, we need to be familiar with how to determine what the problem is asking you to calculate (i.e. probability, expected value, or actual average) and how to apply the lessons learned in the expected means and probability distribution model lessons. First, we will discuss probabilities in a general sense.
A probability is generally defined as the chances or likelihood of an event occurring. It is calculated by identifying two components: the event and the sample space. The event is defined as the favorable outcome or success that we wish to observe. On the other hand, the sample space is defined as the set of all possible outcomes for the event. Mathematically we calculate probabilities by dividing the event by the sample space:
Let's use a simple example: the rolling of a die. We want to know the probability of rolling a one. We know that the sample space is six because there are six sides or outcomes to the die. Also, we know that there is only a single side with a value of one; therefore,
Now, let's convert this into a percentage:
Probabilities expressed in fraction form will have values between zero and one. One indicates that an event will definitely occur, while zero indicates that an event will not occur. Likewise, probabilities expressed as percentages possess values between zero and one hundred percent where probabilities closer to zero are unlikely to occur and those close to one hundred percent are more likely to occur.
According to this logic, we would expect to roll a particular number on a die one out of every six rolls; however, we may roll the same number multiple times or not at all in six rolls. This discrepancy creates a difference between the expected mean and the actual mean. The expected mean is a hypothetical calculation that assumes a very large sample size and no intervening variables (such as differing forces on the roll and changes in the friction of the surface the die is rolled upon between rolls). Under these conditions we can calculate that any number on the die in a "perfect world" should be one out of six. On the other hand the true or actual mean is calculated using "real" date. In these calculations we would roll a die a particular number of times and use it to develop a probability of rolling a particular number. It is important to note that, theoretically, actual means will eventually equal expected means over a large—or near infinite—amount of trials. In other words, over many many rolls we would eventually find that each number on the die has a one in six chance of being rolled.
Now, let's discuss how expected means are determined. The expected mean is calculated using the following formula:
In this equation the variables are identified as the following:
We can illustrate this using an example problem. A list of individuals and the respective size of their motorcycles in cubic inches is provided. If you were to randomly select any one person from the list, then what size would you expect their motorcycle to be?
Let's use this information to solve the problem. In order to solve the motorcycle problem, we need to use the expected mean formula. We will substitute each of the motorcycle owner's engine size with its respective probability and solve.
Each of the values have the same probability of being chosen—one out of eleven. As a result, we can simplify this equation:
Round to the nearest one's place.
Note that we can solve this problem in this simplified manner because all of the people had an equal probability of being chosen. If his or her probabilities differed, then we would need to substitute in each person’s respective probability of being chosen.
Finally, we need to determine how we will discuss how to create a probability distribution graph for a probability model. We will use the following equation to calculate the probabilities to be used in this graphical display:
Remember that in combinations and permutations a combination is calculated using the following formula:
Now, we can write the following formula.
In this formula variables are defined in the following manner:
Let's investigate this standard through the use of an example. Suppose a researcher rolls a die twelve times and notes whether the die rolls on an even or an odd number. If the die is fair (i.e. every number has an equal probability of being rolled or each roll is random), then would the probability distribution graph follow the pattern of a normal distribution? Lets create a table and solve for each variable. The probability of rolling an even number—a two, four, or a six— is three out of six or fifty percent. Likewise, the probability of failure (i.e. rolling an odd number) is three out of six or fifty percent. Next, we need to list the number of successes for each event—variable . The researcher can roll an even number every time he rolls and he may not even roll an even number in all twelve trial. We need to calculate this probability for every possible number of successful events; therefore, the number of successes ranges from zero to twelve. Last, we know that there are a total of twelve trials. We have solved for the probability of each of these variables for every possible number of successes in the trials.
Once this data is tabulated we can graph the probability of rolling an even number. If we look at the graph then we can see if it follows the bell shape curve of a normal distribution.
A bell curve is shaped like the following image:
We can quickly tell that the graph of the probability distribution does follow the shape of a normal or "bell" curve. Now we can use this information to solve the given question.
In the question we are told that the seismologists observed earthquakes. We also know that the probability of an earthquake creating a tsunami is
. Now, we need to find out the probability of three tsunamis hitting the region in the next year. In order to do so, we will use the following equation:
We need to solve for the following probability:
Now, we need to name our variables:
Now, we can substitute in these variables and solve our equation.
Simplify.
Solve and remember to follow the order of operations.
Rewrite in scientific notation.
Example Question #372 : High School: Statistics & Probability
In a particular African coastal region, seismologists studied tsunamis by observing seismic shifts and earthquakes in the South Pacific Ocean. During their study, they were able to note the number of earthquakes as well as the number of resulting tsunamis in this region. They observed earthquakes and found that there was
probability of a tsunami hitting the region in the next year based on previous data. What is the probability that the region will have
tsunamis in the next year?
Cannot be determined
This standard requires us to work backwards in order to deconstruct and solve a problem. At their core, questions in this standard ask us to make approximations about sample statistics while using population parameters and distributions. In other words, these standards use real data to help us approximate values of sample characteristics. It is important to note that these questions—in particular—utilize real data. This means that the data is collected in studies through the observation of real-world situations rather than examples of probabilities calculated through theoretical scenarios.
In these questions, students will be asked to use selections of sample data to calculate probabilities be able to identify expected values; thus, we need to be familiar with how to determine what the problem is asking you to calculate (i.e. probability, expected value, or actual average) and how to apply the lessons learned in the expected means and probability distribution model lessons. First, we will discuss probabilities in a general sense.
A probability is generally defined as the chances or likelihood of an event occurring. It is calculated by identifying two components: the event and the sample space. The event is defined as the favorable outcome or success that we wish to observe. On the other hand, the sample space is defined as the set of all possible outcomes for the event. Mathematically we calculate probabilities by dividing the event by the sample space:
Let's use a simple example: the rolling of a die. We want to know the probability of rolling a one. We know that the sample space is six because there are six sides or outcomes to the die. Also, we know that there is only a single side with a value of one; therefore,
Now, let's convert this into a percentage:
Probabilities expressed in fraction form will have values between zero and one. One indicates that an event will definitely occur, while zero indicates that an event will not occur. Likewise, probabilities expressed as percentages possess values between zero and one hundred percent where probabilities closer to zero are unlikely to occur and those close to one hundred percent are more likely to occur.
According to this logic, we would expect to roll a particular number on a die one out of every six rolls; however, we may roll the same number multiple times or not at all in six rolls. This discrepancy creates a difference between the expected mean and the actual mean. The expected mean is a hypothetical calculation that assumes a very large sample size and no intervening variables (such as differing forces on the roll and changes in the friction of the surface the die is rolled upon between rolls). Under these conditions we can calculate that any number on the die in a "perfect world" should be one out of six. On the other hand the true or actual mean is calculated using "real" date. In these calculations we would roll a die a particular number of times and use it to develop a probability of rolling a particular number. It is important to note that, theoretically, actual means will eventually equal expected means over a large—or near infinite—amount of trials. In other words, over many many rolls we would eventually find that each number on the die has a one in six chance of being rolled.
Now, let's discuss how expected means are determined. The expected mean is calculated using the following formula:
In this equation the variables are identified as the following:
We can illustrate this using an example problem. A list of individuals and the respective size of their motorcycles in cubic inches is provided. If you were to randomly select any one person from the list, then what size would you expect their motorcycle to be?
Let's use this information to solve the problem. In order to solve the motorcycle problem, we need to use the expected mean formula. We will substitute each of the motorcycle owner's engine size with its respective probability and solve.
Each of the values have the same probability of being chosen—one out of eleven. As a result, we can simplify this equation:
Round to the nearest one's place.
Note that we can solve this problem in this simplified manner because all of the people had an equal probability of being chosen. If his or her probabilities differed, then we would need to substitute in each person’s respective probability of being chosen.
Finally, we need to determine how we will discuss how to create a probability distribution graph for a probability model. We will use the following equation to calculate the probabilities to be used in this graphical display:
Remember that in combinations and permutations a combination is calculated using the following formula:
Now, we can write the following formula.
In this formula variables are defined in the following manner:
Let's investigate this standard through the use of an example. Suppose a researcher rolls a die twelve times and notes whether the die rolls on an even or an odd number. If the die is fair (i.e. every number has an equal probability of being rolled or each roll is random), then would the probability distribution graph follow the pattern of a normal distribution? Lets create a table and solve for each variable. The probability of rolling an even number—a two, four, or a six— is three out of six or fifty percent. Likewise, the probability of failure (i.e. rolling an odd number) is three out of six or fifty percent. Next, we need to list the number of successes for each event—variable . The researcher can roll an even number every time he rolls and he may not even roll an even number in all twelve trial. We need to calculate this probability for every possible number of successful events; therefore, the number of successes ranges from zero to twelve. Last, we know that there are a total of twelve trials. We have solved for the probability of each of these variables for every possible number of successes in the trials.
Once this data is tabulated we can graph the probability of rolling an even number. If we look at the graph then we can see if it follows the bell shape curve of a normal distribution.
A bell curve is shaped like the following image:
We can quickly tell that the graph of the probability distribution does follow the shape of a normal or "bell" curve. Now we can use this information to solve the given question.
In the question we are told that the seismologists observed earthquakes. We also know that the probability of an earthquake creating a tsunami is
. Now, we need to find out the probability of three tsunamis hitting the region in the next year. In order to do so, we will use the following equation:
We need to solve for the following probability:
Now, we need to name our variables:
Now, we can substitute in these variables and solve our equation.
Simplify.
Solve and remember to follow the order of operations.
Rewrite in scientific notation.
Example Question #373 : High School: Statistics & Probability
In a particular African coastal region, seismologists studied tsunamis by observing seismic shifts and earthquakes in the South Pacific Ocean. During their study, they were able to note the number of earthquakes as well as the number of resulting tsunamis in this region. They observed earthquakes and found that there was
probability of a tsunami hitting the region in the next year based on previous data. What is the probability that the region will have
tsunamis in the next year?
Cannot be determined
This standard requires us to work backwards in order to deconstruct and solve a problem. At their core, questions in this standard ask us to make approximations about sample statistics while using population parameters and distributions. In other words, these standards use real data to help us approximate values of sample characteristics. It is important to note that these questions—in particular—utilize real data. This means that the data is collected in studies through the observation of real-world situations rather than examples of probabilities calculated through theoretical scenarios.
In these questions, students will be asked to use selections of sample data to calculate probabilities be able to identify expected values; thus, we need to be familiar with how to determine what the problem is asking you to calculate (i.e. probability, expected value, or actual average) and how to apply the lessons learned in the expected means and probability distribution model lessons. First, we will discuss probabilities in a general sense.
A probability is generally defined as the chances or likelihood of an event occurring. It is calculated by identifying two components: the event and the sample space. The event is defined as the favorable outcome or success that we wish to observe. On the other hand, the sample space is defined as the set of all possible outcomes for the event. Mathematically we calculate probabilities by dividing the event by the sample space:
Let's use a simple example: the rolling of a die. We want to know the probability of rolling a one. We know that the sample space is six because there are six sides or outcomes to the die. Also, we know that there is only a single side with a value of one; therefore,
Now, let's convert this into a percentage:
Probabilities expressed in fraction form will have values between zero and one. One indicates that an event will definitely occur, while zero indicates that an event will not occur. Likewise, probabilities expressed as percentages possess values between zero and one hundred percent where probabilities closer to zero are unlikely to occur and those close to one hundred percent are more likely to occur.
According to this logic, we would expect to roll a particular number on a die one out of every six rolls; however, we may roll the same number multiple times or not at all in six rolls. This discrepancy creates a difference between the expected mean and the actual mean. The expected mean is a hypothetical calculation that assumes a very large sample size and no intervening variables (such as differing forces on the roll and changes in the friction of the surface the die is rolled upon between rolls). Under these conditions we can calculate that any number on the die in a "perfect world" should be one out of six. On the other hand the true or actual mean is calculated using "real" date. In these calculations we would roll a die a particular number of times and use it to develop a probability of rolling a particular number. It is important to note that, theoretically, actual means will eventually equal expected means over a large—or near infinite—amount of trials. In other words, over many many rolls we would eventually find that each number on the die has a one in six chance of being rolled.
Now, let's discuss how expected means are determined. The expected mean is calculated using the following formula:
In this equation the variables are identified as the following:
We can illustrate this using an example problem. A list of individuals and the respective size of their motorcycles in cubic inches is provided. If you were to randomly select any one person from the list, then what size would you expect their motorcycle to be?
Let's use this information to solve the problem. In order to solve the motorcycle problem, we need to use the expected mean formula. We will substitute each of the motorcycle owner's engine size with its respective probability and solve.
Each of the values have the same probability of being chosen—one out of eleven. As a result, we can simplify this equation:
Round to the nearest one's place.
Note that we can solve this problem in this simplified manner because all of the people had an equal probability of being chosen. If his or her probabilities differed, then we would need to substitute in each person’s respective probability of being chosen.
Finally, we need to determine how we will discuss how to create a probability distribution graph for a probability model. We will use the following equation to calculate the probabilities to be used in this graphical display:
Remember that in combinations and permutations a combination is calculated using the following formula:
Now, we can write the following formula.
In this formula variables are defined in the following manner:
Let's investigate this standard through the use of an example. Suppose a researcher rolls a die twelve times and notes whether the die rolls on an even or an odd number. If the die is fair (i.e. every number has an equal probability of being rolled or each roll is random), then would the probability distribution graph follow the pattern of a normal distribution? Lets create a table and solve for each variable. The probability of rolling an even number—a two, four, or a six— is three out of six or fifty percent. Likewise, the probability of failure (i.e. rolling an odd number) is three out of six or fifty percent. Next, we need to list the number of successes for each event—variable . The researcher can roll an even number every time he rolls and he may not even roll an even number in all twelve trial. We need to calculate this probability for every possible number of successful events; therefore, the number of successes ranges from zero to twelve. Last, we know that there are a total of twelve trials. We have solved for the probability of each of these variables for every possible number of successes in the trials.
Once this data is tabulated we can graph the probability of rolling an even number. If we look at the graph then we can see if it follows the bell shape curve of a normal distribution.
A bell curve is shaped like the following image:
We can quickly tell that the graph of the probability distribution does follow the shape of a normal or "bell" curve. Now we can use this information to solve the given question.
In the question we are told that the seismologists observed earthquakes. We also know that the probability of an earthquake creating a tsunami is
. Now, we need to find out the probability of three tsunamis hitting the region in the next year. In order to do so, we will use the following equation:
We need to solve for the following probability:
Now, we need to name our variables:
Now, we can substitute in these variables and solve our equation.
Simplify.
Solve and remember to follow the order of operations.
Rewrite in scientific notation.
Example Question #3 : Develop Probability Distribution For Random Variable With Empirically Assigned Probabilities: Ccss.Math.Content.Hss Md.A.4
In a particular African coastal region, seismologists studied tsunamis by observing seismic shifts and earthquakes in the South Pacific Ocean. During their study, they were able to note the number of earthquakes as well as the number of resulting tsunamis in this region. They observed earthquakes and found that there was
probability of a tsunami hitting the region in the next year based on previous data. What is the probability that the region will have
tsunamis in the next year?
Cannot be determined
This standard requires us to work backwards in order to deconstruct and solve a problem. At their core, questions in this standard ask us to make approximations about sample statistics while using population parameters and distributions. In other words, these standards use real data to help us approximate values of sample characteristics. It is important to note that these questions—in particular—utilize real data. This means that the data is collected in studies through the observation of real-world situations rather than examples of probabilities calculated through theoretical scenarios.
In these questions, students will be asked to use selections of sample data to calculate probabilities be able to identify expected values; thus, we need to be familiar with how to determine what the problem is asking you to calculate (i.e. probability, expected value, or actual average) and how to apply the lessons learned in the expected means and probability distribution model lessons. First, we will discuss probabilities in a general sense.
A probability is generally defined as the chances or likelihood of an event occurring. It is calculated by identifying two components: the event and the sample space. The event is defined as the favorable outcome or success that we wish to observe. On the other hand, the sample space is defined as the set of all possible outcomes for the event. Mathematically we calculate probabilities by dividing the event by the sample space:
Let's use a simple example: the rolling of a die. We want to know the probability of rolling a one. We know that the sample space is six because there are six sides or outcomes to the die. Also, we know that there is only a single side with a value of one; therefore,
Now, let's convert this into a percentage:
Probabilities expressed in fraction form will have values between zero and one. One indicates that an event will definitely occur, while zero indicates that an event will not occur. Likewise, probabilities expressed as percentages possess values between zero and one hundred percent where probabilities closer to zero are unlikely to occur and those close to one hundred percent are more likely to occur.
According to this logic, we would expect to roll a particular number on a die one out of every six rolls; however, we may roll the same number multiple times or not at all in six rolls. This discrepancy creates a difference between the expected mean and the actual mean. The expected mean is a hypothetical calculation that assumes a very large sample size and no intervening variables (such as differing forces on the roll and changes in the friction of the surface the die is rolled upon between rolls). Under these conditions we can calculate that any number on the die in a "perfect world" should be one out of six. On the other hand the true or actual mean is calculated using "real" date. In these calculations we would roll a die a particular number of times and use it to develop a probability of rolling a particular number. It is important to note that, theoretically, actual means will eventually equal expected means over a large—or near infinite—amount of trials. In other words, over many many rolls we would eventually find that each number on the die has a one in six chance of being rolled.
Now, let's discuss how expected means are determined. The expected mean is calculated using the following formula:
In this equation the variables are identified as the following:
We can illustrate this using an example problem. A list of individuals and the respective size of their motorcycles in cubic inches is provided. If you were to randomly select any one person from the list, then what size would you expect their motorcycle to be?
Let's use this information to solve the problem. In order to solve the motorcycle problem, we need to use the expected mean formula. We will substitute each of the motorcycle owner's engine size with its respective probability and solve.
Each of the values have the same probability of being chosen—one out of eleven. As a result, we can simplify this equation:
Round to the nearest one's place.
Note that we can solve this problem in this simplified manner because all of the people had an equal probability of being chosen. If his or her probabilities differed, then we would need to substitute in each person’s respective probability of being chosen.
Finally, we need to determine how we will discuss how to create a probability distribution graph for a probability model. We will use the following equation to calculate the probabilities to be used in this graphical display:
Remember that in combinations and permutations a combination is calculated using the following formula:
Now, we can write the following formula.
In this formula variables are defined in the following manner:
Let's investigate this standard through the use of an example. Suppose a researcher rolls a die twelve times and notes whether the die rolls on an even or an odd number. If the die is fair (i.e. every number has an equal probability of being rolled or each roll is random), then would the probability distribution graph follow the pattern of a normal distribution? Lets create a table and solve for each variable. The probability of rolling an even number—a two, four, or a six— is three out of six or fifty percent. Likewise, the probability of failure (i.e. rolling an odd number) is three out of six or fifty percent. Next, we need to list the number of successes for each event—variable . The researcher can roll an even number every time he rolls and he may not even roll an even number in all twelve trial. We need to calculate this probability for every possible number of successful events; therefore, the number of successes ranges from zero to twelve. Last, we know that there are a total of twelve trials. We have solved for the probability of each of these variables for every possible number of successes in the trials.
Once this data is tabulated we can graph the probability of rolling an even number. If we look at the graph then we can see if it follows the bell shape curve of a normal distribution.
A bell curve is shaped like the following image:
We can quickly tell that the graph of the probability distribution does follow the shape of a normal or "bell" curve. Now we can use this information to solve the given question.
In the question we are told that the seismologists observed earthquakes. We also know that the probability of an earthquake creating a tsunami is
. Now, we need to find out the probability of three tsunamis hitting the region in the next year. In order to do so, we will use the following equation:
We need to solve for the following probability:
Now, we need to name our variables:
Now, we can substitute in these variables and solve our equation.
Simplify.
Solve and remember to follow the order of operations.
Rewrite in scientific notation.
Example Question #374 : High School: Statistics & Probability
In a particular African coastal region, seismologists studied tsunamis by observing seismic shifts and earthquakes in the South Pacific Ocean. During their study, they were able to note the number of earthquakes as well as the number of resulting tsunamis in this region. They observed earthquakes and found that there was
probability of a tsunami hitting the region in the next year based on previous data. What is the probability that the region will have
tsunamis in the next year?
Cannot be determined
This standard requires us to work backwards in order to deconstruct and solve a problem. At their core, questions in this standard ask us to make approximations about sample statistics while using population parameters and distributions. In other words, these standards use real data to help us approximate values of sample characteristics. It is important to note that these questions—in particular—utilize real data. This means that the data is collected in studies through the observation of real-world situations rather than examples of probabilities calculated through theoretical scenarios.
In these questions, students will be asked to use selections of sample data to calculate probabilities be able to identify expected values; thus, we need to be familiar with how to determine what the problem is asking you to calculate (i.e. probability, expected value, or actual average) and how to apply the lessons learned in the expected means and probability distribution model lessons. First, we will discuss probabilities in a general sense.
A probability is generally defined as the chances or likelihood of an event occurring. It is calculated by identifying two components: the event and the sample space. The event is defined as the favorable outcome or success that we wish to observe. On the other hand, the sample space is defined as the set of all possible outcomes for the event. Mathematically we calculate probabilities by dividing the event by the sample space:
Let's use a simple example: the rolling of a die. We want to know the probability of rolling a one. We know that the sample space is six because there are six sides or outcomes to the die. Also, we know that there is only a single side with a value of one; therefore,
Now, let's convert this into a percentage:
Probabilities expressed in fraction form will have values between zero and one. One indicates that an event will definitely occur, while zero indicates that an event will not occur. Likewise, probabilities expressed as percentages possess values between zero and one hundred percent where probabilities closer to zero are unlikely to occur and those close to one hundred percent are more likely to occur.
According to this logic, we would expect to roll a particular number on a die one out of every six rolls; however, we may roll the same number multiple times or not at all in six rolls. This discrepancy creates a difference between the expected mean and the actual mean. The expected mean is a hypothetical calculation that assumes a very large sample size and no intervening variables (such as differing forces on the roll and changes in the friction of the surface the die is rolled upon between rolls). Under these conditions we can calculate that any number on the die in a "perfect world" should be one out of six. On the other hand the true or actual mean is calculated using "real" date. In these calculations we would roll a die a particular number of times and use it to develop a probability of rolling a particular number. It is important to note that, theoretically, actual means will eventually equal expected means over a large—or near infinite—amount of trials. In other words, over many many rolls we would eventually find that each number on the die has a one in six chance of being rolled.
Now, let's discuss how expected means are determined. The expected mean is calculated using the following formula:
In this equation the variables are identified as the following:
We can illustrate this using an example problem. A list of individuals and the respective size of their motorcycles in cubic inches is provided. If you were to randomly select any one person from the list, then what size would you expect their motorcycle to be?
Let's use this information to solve the problem. In order to solve the motorcycle problem, we need to use the expected mean formula. We will substitute each of the motorcycle owner's engine size with its respective probability and solve.
Each of the values have the same probability of being chosen—one out of eleven. As a result, we can simplify this equation:
Round to the nearest one's place.
Note that we can solve this problem in this simplified manner because all of the people had an equal probability of being chosen. If his or her probabilities differed, then we would need to substitute in each person’s respective probability of being chosen.
Finally, we need to determine how we will discuss how to create a probability distribution graph for a probability model. We will use the following equation to calculate the probabilities to be used in this graphical display:
Remember that in combinations and permutations a combination is calculated using the following formula:
Now, we can write the following formula.
In this formula variables are defined in the following manner:
Let's investigate this standard through the use of an example. Suppose a researcher rolls a die twelve times and notes whether the die rolls on an even or an odd number. If the die is fair (i.e. every number has an equal probability of being rolled or each roll is random), then would the probability distribution graph follow the pattern of a normal distribution? Lets create a table and solve for each variable. The probability of rolling an even number—a two, four, or a six— is three out of six or fifty percent. Likewise, the probability of failure (i.e. rolling an odd number) is three out of six or fifty percent. Next, we need to list the number of successes for each event—variable . The researcher can roll an even number every time he rolls and he may not even roll an even number in all twelve trial. We need to calculate this probability for every possible number of successful events; therefore, the number of successes ranges from zero to twelve. Last, we know that there are a total of twelve trials. We have solved for the probability of each of these variables for every possible number of successes in the trials.
Once this data is tabulated we can graph the probability of rolling an even number. If we look at the graph then we can see if it follows the bell shape curve of a normal distribution.
A bell curve is shaped like the following image:
We can quickly tell that the graph of the probability distribution does follow the shape of a normal or "bell" curve. Now we can use this information to solve the given question.
In the question we are told that the seismologists observed earthquakes. We also know that the probability of an earthquake creating a tsunami is
. Now, we need to find out the probability of three tsunamis hitting the region in the next year. In order to do so, we will use the following equation:
We need to solve for the following probability:
Now, we need to name our variables:
Now, we can substitute in these variables and solve our equation.
Simplify.
Solve and remember to follow the order of operations.
Rewrite in scientific notation.
Example Question #375 : High School: Statistics & Probability
In a particular African coastal region, seismologists studied tsunamis by observing seismic shifts and earthquakes in the South Pacific Ocean. During their study, they were able to note the number of earthquakes as well as the number of resulting tsunamis in this region. They observed earthquakes and found that there was
probability of a tsunami hitting the region in the next year based on previous data. What is the probability that the region will have
tsunamis in the next year?
Cannot be determined
This standard requires us to work backwards in order to deconstruct and solve a problem. At their core, questions in this standard ask us to make approximations about sample statistics while using population parameters and distributions. In other words, these standards use real data to help us approximate values of sample characteristics. It is important to note that these questions—in particular—utilize real data. This means that the data is collected in studies through the observation of real-world situations rather than examples of probabilities calculated through theoretical scenarios.
In these questions, students will be asked to use selections of sample data to calculate probabilities be able to identify expected values; thus, we need to be familiar with how to determine what the problem is asking you to calculate (i.e. probability, expected value, or actual average) and how to apply the lessons learned in the expected means and probability distribution model lessons. First, we will discuss probabilities in a general sense.
A probability is generally defined as the chances or likelihood of an event occurring. It is calculated by identifying two components: the event and the sample space. The event is defined as the favorable outcome or success that we wish to observe. On the other hand, the sample space is defined as the set of all possible outcomes for the event. Mathematically we calculate probabilities by dividing the event by the sample space:
Let's use a simple example: the rolling of a die. We want to know the probability of rolling a one. We know that the sample space is six because there are six sides or outcomes to the die. Also, we know that there is only a single side with a value of one; therefore,
Now, let's convert this into a percentage:
Probabilities expressed in fraction form will have values between zero and one. One indicates that an event will definitely occur, while zero indicates that an event will not occur. Likewise, probabilities expressed as percentages possess values between zero and one hundred percent where probabilities closer to zero are unlikely to occur and those close to one hundred percent are more likely to occur.
According to this logic, we would expect to roll a particular number on a die one out of every six rolls; however, we may roll the same number multiple times or not at all in six rolls. This discrepancy creates a difference between the expected mean and the actual mean. The expected mean is a hypothetical calculation that assumes a very large sample size and no intervening variables (such as differing forces on the roll and changes in the friction of the surface the die is rolled upon between rolls). Under these conditions we can calculate that any number on the die in a "perfect world" should be one out of six. On the other hand the true or actual mean is calculated using "real" date. In these calculations we would roll a die a particular number of times and use it to develop a probability of rolling a particular number. It is important to note that, theoretically, actual means will eventually equal expected means over a large—or near infinite—amount of trials. In other words, over many many rolls we would eventually find that each number on the die has a one in six chance of being rolled.
Now, let's discuss how expected means are determined. The expected mean is calculated using the following formula:
In this equation the variables are identified as the following:
We can illustrate this using an example problem. A list of individuals and the respective size of their motorcycles in cubic inches is provided. If you were to randomly select any one person from the list, then what size would you expect their motorcycle to be?
Let's use this information to solve the problem. In order to solve the motorcycle problem, we need to use the expected mean formula. We will substitute each of the motorcycle owner's engine size with its respective probability and solve.
Each of the values have the same probability of being chosen—one out of eleven. As a result, we can simplify this equation:
Round to the nearest one's place.
Note that we can solve this problem in this simplified manner because all of the people had an equal probability of being chosen. If his or her probabilities differed, then we would need to substitute in each person’s respective probability of being chosen.
Finally, we need to determine how we will discuss how to create a probability distribution graph for a probability model. We will use the following equation to calculate the probabilities to be used in this graphical display:
Remember that in combinations and permutations a combination is calculated using the following formula:
Now, we can write the following formula.
In this formula variables are defined in the following manner:
Let's investigate this standard through the use of an example. Suppose a researcher rolls a die twelve times and notes whether the die rolls on an even or an odd number. If the die is fair (i.e. every number has an equal probability of being rolled or each roll is random), then would the probability distribution graph follow the pattern of a normal distribution? Lets create a table and solve for each variable. The probability of rolling an even number—a two, four, or a six— is three out of six or fifty percent. Likewise, the probability of failure (i.e. rolling an odd number) is three out of six or fifty percent. Next, we need to list the number of successes for each event—variable . The researcher can roll an even number every time he rolls and he may not even roll an even number in all twelve trial. We need to calculate this probability for every possible number of successful events; therefore, the number of successes ranges from zero to twelve. Last, we know that there are a total of twelve trials. We have solved for the probability of each of these variables for every possible number of successes in the trials.
Once this data is tabulated we can graph the probability of rolling an even number. If we look at the graph then we can see if it follows the bell shape curve of a normal distribution.
A bell curve is shaped like the following image:
We can quickly tell that the graph of the probability distribution does follow the shape of a normal or "bell" curve. Now we can use this information to solve the given question.
In the question we are told that the seismologists observed earthquakes. We also know that the probability of an earthquake creating a tsunami is
. Now, we need to find out the probability of three tsunamis hitting the region in the next year. In order to do so, we will use the following equation:
We need to solve for the following probability:
Now, we need to name our variables:
Now, we can substitute in these variables and solve our equation.
Simplify.
Solve and remember to follow the order of operations.
Rewrite in scientific notation.
Example Question #376 : High School: Statistics & Probability
In a particular African coastal region, seismologists studied tsunamis by observing seismic shifts and earthquakes in the South Pacific Ocean. During their study, they were able to note the number of earthquakes as well as the number of resulting tsunamis in this region. They observed earthquakes and found that there was
probability of a tsunami hitting the region in the next year based on previous data. What is the probability that the region will have
tsunamis in the next year?
Cannot be determined
This standard requires us to work backwards in order to deconstruct and solve a problem. At their core, questions in this standard ask us to make approximations about sample statistics while using population parameters and distributions. In other words, these standards use real data to help us approximate values of sample characteristics. It is important to note that these questions—in particular—utilize real data. This means that the data is collected in studies through the observation of real-world situations rather than examples of probabilities calculated through theoretical scenarios.
In these questions, students will be asked to use selections of sample data to calculate probabilities be able to identify expected values; thus, we need to be familiar with how to determine what the problem is asking you to calculate (i.e. probability, expected value, or actual average) and how to apply the lessons learned in the expected means and probability distribution model lessons. First, we will discuss probabilities in a general sense.
A probability is generally defined as the chances or likelihood of an event occurring. It is calculated by identifying two components: the event and the sample space. The event is defined as the favorable outcome or success that we wish to observe. On the other hand, the sample space is defined as the set of all possible outcomes for the event. Mathematically we calculate probabilities by dividing the event by the sample space:
Let's use a simple example: the rolling of a die. We want to know the probability of rolling a one. We know that the sample space is six because there are six sides or outcomes to the die. Also, we know that there is only a single side with a value of one; therefore,
Now, let's convert this into a percentage:
Probabilities expressed in fraction form will have values between zero and one. One indicates that an event will definitely occur, while zero indicates that an event will not occur. Likewise, probabilities expressed as percentages possess values between zero and one hundred percent where probabilities closer to zero are unlikely to occur and those close to one hundred percent are more likely to occur.
According to this logic, we would expect to roll a particular number on a die one out of every six rolls; however, we may roll the same number multiple times or not at all in six rolls. This discrepancy creates a difference between the expected mean and the actual mean. The expected mean is a hypothetical calculation that assumes a very large sample size and no intervening variables (such as differing forces on the roll and changes in the friction of the surface the die is rolled upon between rolls). Under these conditions we can calculate that any number on the die in a "perfect world" should be one out of six. On the other hand the true or actual mean is calculated using "real" date. In these calculations we would roll a die a particular number of times and use it to develop a probability of rolling a particular number. It is important to note that, theoretically, actual means will eventually equal expected means over a large—or near infinite—amount of trials. In other words, over many many rolls we would eventually find that each number on the die has a one in six chance of being rolled.
Now, let's discuss how expected means are determined. The expected mean is calculated using the following formula:
In this equation the variables are identified as the following:
We can illustrate this using an example problem. A list of individuals and the respective size of their motorcycles in cubic inches is provided. If you were to randomly select any one person from the list, then what size would you expect their motorcycle to be?
Let's use this information to solve the problem. In order to solve the motorcycle problem, we need to use the expected mean formula. We will substitute each of the motorcycle owner's engine size with its respective probability and solve.
Each of the values have the same probability of being chosen—one out of eleven. As a result, we can simplify this equation:
Round to the nearest one's place.
Note that we can solve this problem in this simplified manner because all of the people had an equal probability of being chosen. If his or her probabilities differed, then we would need to substitute in each person’s respective probability of being chosen.
Finally, we need to determine how we will discuss how to create a probability distribution graph for a probability model. We will use the following equation to calculate the probabilities to be used in this graphical display:
Remember that in combinations and permutations a combination is calculated using the following formula:
Now, we can write the following formula.
In this formula variables are defined in the following manner:
Let's investigate this standard through the use of an example. Suppose a researcher rolls a die twelve times and notes whether the die rolls on an even or an odd number. If the die is fair (i.e. every number has an equal probability of being rolled or each roll is random), then would the probability distribution graph follow the pattern of a normal distribution? Lets create a table and solve for each variable. The probability of rolling an even number—a two, four, or a six— is three out of six or fifty percent. Likewise, the probability of failure (i.e. rolling an odd number) is three out of six or fifty percent. Next, we need to list the number of successes for each event—variable . The researcher can roll an even number every time he rolls and he may not even roll an even number in all twelve trial. We need to calculate this probability for every possible number of successful events; therefore, the number of successes ranges from zero to twelve. Last, we know that there are a total of twelve trials. We have solved for the probability of each of these variables for every possible number of successes in the trials.
Once this data is tabulated we can graph the probability of rolling an even number. If we look at the graph then we can see if it follows the bell shape curve of a normal distribution.
A bell curve is shaped like the following image:
We can quickly tell that the graph of the probability distribution does follow the shape of a normal or "bell" curve. Now we can use this information to solve the given question.
In the question we are told that the seismologists observed earthquakes. We also know that the probability of an earthquake creating a tsunami is
. Now, we need to find out the probability of three tsunamis hitting the region in the next year. In order to do so, we will use the following equation:
We need to solve for the following probability:
Now, we need to name our variables:
Now, we can substitute in these variables and solve our equation.
Simplify.
Solve and remember to follow the order of operations.
Rewrite in scientific notation.
All Common Core: High School - Statistics and Probability Resources
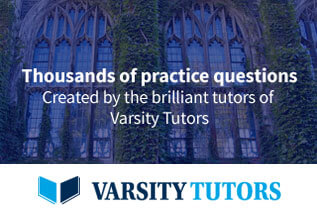