All Common Core: High School - Statistics and Probability Resources
Example Questions
Example Question #1 : Estimate Population Mean And Margin Of Error: Ccss.Math.Content.Hss Ic.B.4
A car manufacturer wants to produce a sports car that has an average quarter mile run time of:
Researchers decide to randomly sample two hundred cars off of the stock production line. They find that these cars' mean quarter mile run time is as follows:
Is it reasonable to say that the car performs to the manufacturer's specifications?
Cannot be determined
No
Yes
Yes
In order to solve this question, we need to learn how to infer population parameters from sample statistics. We will do this by reviewing the definitions of parameters and statistics, recalling the processes associated with the scientific method, and by interrogating the information in the problem.
First let's discuss what is meant by the term population. In statistics, a "population" is described as the entire group that is to be studied. An example of a population in the natural sciences would be every giant panda of the species Ailuropoda melanoleuca in the wild (1864 individuals according to the World Wildlife Foundation)—not captivity. Now, let's identify what is meant by the term population parameter. A "population parameter" is a statistic that is found by sampling the entire population. For example, the mean weight of the entire wild population of giant pandas in the world would be an example of a population parameter (i.e. the mean weight of all 1864 pandas). Next, we will discuss sample populations and statistics.
A "sample" is the subset of a population that is being studied. For example, researchers for a university want to study giant pandas in the wild but can only access a group of 100 pandas sampled in Sichuan, China. Data collected from this particular study would be known as a sample statistic (e.g. the mean weight of pandas in the Sichuan region). It is important to note that the external validity of some sample statistics are hindered. The external validity of a statistic is its ability to be applied to other samples and remain valid. If locals fed pandas in the Sichuan region, then their mean weight may be greater than those of the southern or northern regions. In this instance, the mean would not be representative of other populations of giant pandas.
Last, we should note that certain sample populations are better than others at predicting population parameters. A population parameter can be considered to be the true statistic of a given population while a sample statistic is only an estimate of a part or subset of the population. Simple random samples are good predictors of population parameters and can be used to estimate them. They are collected when every member in a population has an equal chance of being chosen (e.g. randomly selecting 100 of the 1864 pandas in the world).
Next, we shall observe the steps associated with the scientific method.
In this method, researchers observe a phenomenon and develop a tentative explanation for it. Afterwards, they develop an experiment, which assigns subjects or variables to control and treatment groups. Experiments measure the change that an independent variable (i.e. treatment) has on a dependent variable (i.e. subject or phenomena under investigation). Scientists repeat or replicate these experiments multiple times in order gather data for statistical analysis. Scientists analyze the data and then use this evidence to either support or refute a hypothesis. Hypotheses can only be supported or refuted: never proven. This is because scientific investigation can only gather evidence for or against a particular phenomenon. Even theories such as gravity and natural selection have not been proven—they are simply supported by an almost endless amount of scientific evidence.
Last, let's use this information to solve the following problem:
A car manufacturer wants to produce a sports car that has the following average quarter mile run time:
Researchers decide to randomly sample two hundred cars off of the stock production line. They find that these cars' quarter mile run mean time is as follows:
Is it reasonable to say that the car performs to the manufacturer's specifications?
The researchers found that the mean time of two hundred cars was:
According to this sample statistic, it is reasonable to assume that the car can and will perform to the specifications of the manufacturer. This is because the calculated mean run time is only slightly above the desired average quarter mile run time. The time that was calculated in the experiment represents the test statistic, while the manufacturer's desired quarter mile run time represents the population parameter. The statistic represents a single experiment used to test a hypothesis that stated: the new car has an average quarter mile run time of
This hypothesis was tested by a single experiment that measured the quarter mile run time of two hundred stock cars. Replication of this experiment would gather means of multiple samples. If the researchers were able to gather a census of the quarter mile run time of the population, then they could create a standard curve:
A standard distribution represents a distribution of the means; furthermore, its mean (i.e. the mean of the means) is hypothetically equal to the population parameter mean.
It is costly and unlikely that a researcher will be able to take a census of all the instances of a particular phenomenon. As a result, many researchers will take a limited sample and fit a standard curve to it in order to gather a representative estimate of a populations mean—in this case quarter mile run times. In this example, researchers could gather the mean of one hundred experiments and create the following graph:
We can see in this graph that the sample statistic——hovers around the center—mean of the means—of the graph; therefore, it is reasonable to assume that this statistic supports the hypothesis that stated that the car could meet designer specifications.
Example Question #2 : Estimate Population Mean And Margin Of Error: Ccss.Math.Content.Hss Ic.B.4
A car manufacturer wants to produce a sports car that has an average quarter mile run time of:
Researchers decide to randomly sample two hundred cars off of the stock production line. They find that these cars' mean quarter mile run time is as follows:
Is it reasonable to say that the car performs to the manufacturer's specifications?
Cannot be determined
Yes
No
Yes
In order to solve this question, we need to learn how to infer population parameters from sample statistics. We will do this by reviewing the definitions of parameters and statistics, recalling the processes associated with the scientific method, and by interrogating the information in the problem.
First, let's discuss what is meant by the term population. In statistics, a "population" is described as the entire group that is to be studied. An example of a population in the natural sciences would be every giant panda of the species Ailuropoda melanoleuca in the wild (1864 individuals according to the World Wildlife Foundation)—not captivity. Now, let's identify what is meant by the term population parameter. A "population parameter" is a statistic that is found by sampling the entire population. For example, the mean weight of the entire wild population of giant pandas in the world would be an example of a population parameter (i.e. the mean weight of all 1864 pandas). Next, we will discuss sample populations and statistics.
A "sample" is the subset of a population that is being studied. For example, researchers for a university want to study giant pandas in the wild but can only access a group of 100 pandas sampled in Sichuan, China. Data collected from this particular study would be known as a sample statistic (e.g. the mean weight of pandas in the Sichuan region). It is important to note that the external validity of some sample statistics are hindered. The external validity of a statistic is its ability to be applied to other samples and remain valid. If locals fed pandas in the Sichuan region, then their mean weight may be greater than those of the southern or northern regions. In this instance, the mean would not be representative of other populations of giant pandas.
Last, we should note that certain sample populations are better than others at predicting population parameters. A population parameter can be considered to be the true statistic of a given population while a sample statistic is only an estimate of a part or subset of the population. Simple random samples are good predictors of population parameters and can be used to estimate them. They are collected when every member in a population has an equal chance of being chosen (e.g. randomly selecting 100 of the 1864 pandas in the world).
Next, we shall observe the steps associated with the scientific method.
In this method, researchers observe a phenomenon and develop a tentative explanation for it. Afterwards, they develop an experiment, which assigns subjects or variables to control and treatment groups. Experiments measure the change that an independent variable (i.e. treatment) has on a dependent variable (i.e. subject or phenomena under investigation). Scientists repeat or replicate these experiments multiple times in order gather data for statistical analysis. Scientists analyze the data and then use this evidence to either support or refute a hypothesis. Hypotheses can only be supported or refuted: never proven. This is because scientific investigation can only gather evidence for or against a particular phenomenon. Even theories such as gravity and natural selection have not been proven—they are simply supported by an almost endless amount of scientific evidence.
Last, let's use this information to solve the following problem:
A car manufacturer wants to produce a sports car that has the following average quarter mile run time:
Researchers decide to randomly sample two hundred cars off of the stock production line. They find that these cars' quarter mile run mean time is as follows:
Is it reasonable to say that the car performs to the manufacturer's specifications?
The researchers found that the mean time of two hundred cars was:
According to this sample statistic, it is reasonable to assume that the car can and will perform to the specifications of the manufacturer. This is because the calculated mean run time is only slightly above the desired average quarter mile run time. The time that was calculated in the experiment represents the test statistic, while the manufacturer's desired quarter mile run time represents the population parameter. The statistic represents a single experiment used to test a hypothesis that stated: the new car has an average quarter mile run time of
This hypothesis was tested by a single experiment that measured the quarter mile run time of two hundred stock cars. Replication of this experiment would gather means of multiple samples. If the researchers were able to gather a census of the quarter mile run time of the population, then they could create a standard curve:
A standard distribution represents a distribution of the means; furthermore, its mean (i.e. the mean of the means) is hypothetically equal to the population parameter mean.
It is costly and unlikely that a researcher will be able to take a census of all the instances of a particular phenomenon. As a result, many researchers will take a limited sample and fit a standard curve to it in order to gather a representative estimate of a populations mean—in this case quarter mile run times. In this example, researchers could gather the mean of one hundred experiments and create the following graph:
We can see in this graph that the sample statistic hovers around the center—mean of the means—of the graph; therefore, it is reasonable to assume that this statistic supports the hypothesis that stated that the car could meet designer specifications.
Example Question #3 : Estimate Population Mean And Margin Of Error: Ccss.Math.Content.Hss Ic.B.4
A car manufacturer wants to produce a sports car that has an average quarter mile run time of:
Researchers decide to randomly sample two hundred cars off of the stock production line. They find that these cars' mean quarter mile run time is as follows:
Is it reasonable to say that the car performs to the manufacturer's specifications?
Yes
Cannot be determined
No
No
In order to solve this question, we need to learn how to infer population parameters from sample statistics. We will do this by reviewing the definitions of parameters and statistics, recalling the processes associated with the scientific method, and by interrogating the information in the problem.
First, let's discuss what is meant by the term population. In statistics, a "population" is described as the entire group that is to be studied. An example of a population in the natural sciences would be every giant panda of the species Ailuropoda melanoleuca in the wild (1864 individuals according to the World Wildlife Foundation)—not captivity. Now, let's identify what is meant by the term population parameter. A "population parameter" is a statistic that is found by sampling the entire population. For example, the mean weight of the entire wild population of giant pandas in the world would be an example of a population parameter (i.e. the mean weight of all 1864 pandas). Next, we will discuss sample populations and statistics.
A "sample" is the subset of a population that is being studied. For example, researchers for a university want to study giant pandas in the wild but can only access a group of 100 pandas sampled in Sichuan, China. Data collected from this particular study would be known as a sample statistic (e.g. the mean weight of pandas in the Sichuan region). It is important to note that the external validity of some sample statistics are hindered. The external validity of a statistic is its ability to be applied to other samples and remain valid. If locals fed pandas in the Sichuan region, then their mean weight may be greater than those of the southern or northern regions. In this instance, the mean would not be representative of other populations of giant pandas.
Last, we should note that certain sample populations are better than others at predicting population parameters. A population parameter can be considered to be the true statistic of a given population while a sample statistic is only an estimate of a part or subset of the population. Simple random samples are good predictors of population parameters and can be used to estimate them. They are collected when every member in a population has an equal chance of being chosen (e.g. randomly selecting 100 of the 1864 pandas in the world).
Next, we shall observe the steps associated with the scientific method.
In this method, researchers observe a phenomenon and develop a tentative explanation for it. Afterwards, they develop an experiment, which assigns subjects or variables to control and treatment groups. Experiments measure the change that an independent variable (i.e. treatment) has on a dependent variable (i.e. subject or phenomena under investigation). Scientists repeat or replicate these experiments multiple times in order gather data for statistical analysis. Scientists analyze the data and then use this evidence to either support or refute a hypothesis. Hypotheses can only be supported or refuted: never proven. This is because scientific investigation can only gather evidence for or against a particular phenomenon. Even theories such as gravity and natural selection have not been proven—they are simply supported by an almost endless amount of scientific evidence.
Last, let's use this information to solve the following problem:
A car manufacturer wants to produce a sports car that has the following average quarter mile run time:
Researchers decide to randomly sample two hundred cars off of the stock production line. They find that these cars' quarter mile run mean time is as follows:
Is it reasonable to say that the car performs to the manufacturer's specifications?
The researchers found that the mean time of two hundred cars was:
According to this sample statistic, it is not reasonable to assume that the car can perform to the specifications of the manufacturer. This is because the calculated mean run time is far above the desired average quarter mile run time. The time that was calculated in the experiment represents the test statistic, while the manufacturer's desired quarter mile run time represents the population parameter. The statistic represents a single experiment used to test a hypothesis that stated: the new car has an average quarter mile run time of
This hypothesis was tested by a single experiment that measured the quarter mile run time of two hundred stock cars. Replication of this experiment would gather means of multiple samples. If the researchers were able to gather a census of the quarter mile run time of the population, then they could create a standard curve:
A standard distribution represents a distribution of the means; furthermore, its mean (i.e. the mean of the means) is hypothetically equal to the population parameter mean.
It is costly and unlikely that a researcher will be able to take a census of all the instances of a particular phenomenon. As a result, many researchers will take a limited sample and fit a standard curve to it in order to gather a representative estimate of a populations mean—in this case quarter mile run times. In this example, researchers could gather the mean of one hundred experiments and create the following graph:
We can see in this graph that the sample statistic doesn't hover around the center—mean of the means—of the graph; therefore, it is not reasonable to assume that this statistic supports the hypothesis that stated that the car could meet designer specifications.
Example Question #1 : Estimate Population Mean And Margin Of Error: Ccss.Math.Content.Hss Ic.B.4
A car manufacturer wants to produce a sports car that has an average quarter mile run time of:
Researchers decide to randomly sample two hundred cars off of the stock production line. They find that these cars' mean quarter mile run time is as follows:
Is it reasonable to say that the car performs to the manufacturer's specifications?
No
Yes
Cannot be determined
No
In order to solve this question, we need to learn how to infer population parameters from sample statistics. We will do this by reviewing the definitions of parameters and statistics, recalling the processes associated with the scientific method, and by interrogating the information in the problem.
First, let's discuss what is meant by the term population. In statistics, a "population" is described as the entire group that is to be studied. An example of a population in the natural sciences would be every giant panda of the species Ailuropoda melanoleuca in the wild (1864 individuals according to the World Wildlife Foundation)—not captivity. Now, let's identify what is meant by the term population parameter. A "population parameter" is a statistic that is found by sampling the entire population. For example, the mean weight of the entire wild population of giant pandas in the world would be an example of a population parameter (i.e. the mean weight of all 1864 pandas). Next, we will discuss sample populations and statistics.
A "sample" is the subset of a population that is being studied. For example, researchers for a university want to study giant pandas in the wild but can only access a group of 100 pandas sampled in Sichuan, China. Data collected from this particular study would be known as a sample statistic (e.g. the mean weight of pandas in the Sichuan region). It is important to note that the external validity of some sample statistics are hindered. The external validity of a statistic is its ability to be applied to other samples and remain valid. If locals fed pandas in the Sichuan region, then their mean weight may be greater than those of the southern or northern regions. In this instance, the mean would not be representative of other populations of giant pandas.
Last, we should note that certain sample populations are better than others at predicting population parameters. A population parameter can be considered to be the true statistic of a given population while a sample statistic is only an estimate of a part or subset of the population. Simple random samples are good predictors of population parameters and can be used to estimate them. They are collected when every member in a population has an equal chance of being chosen (e.g. randomly selecting 100 of the 1864 pandas in the world).
Next, we shall observe the steps associated with the scientific method.
In this method, researchers observe a phenomenon and develop a tentative explanation for it. Afterwards, they develop an experiment, which assigns subjects or variables to control and treatment groups. Experiments measure the change that an independent variable (i.e. treatment) has on a dependent variable (i.e. subject or phenomena under investigation). Scientists repeat or replicate these experiments multiple times in order gather data for statistical analysis. Scientists analyze the data and then use this evidence to either support or refute a hypothesis. Hypotheses can only be supported or refuted: never proven. This is because scientific investigation can only gather evidence for or against a particular phenomenon. Even theories such as gravity and natural selection have not been proven—they are simply supported by an almost endless amount of scientific evidence.
Last, let's use this information to solve the following problem:
A car manufacturer wants to produce a sports car that has the following average quarter mile run time:
Researchers decide to randomly sample two hundred cars off of the stock production line. They find that these cars' quarter mile run mean time is as follows:
Is it reasonable to say that the car performs to the manufacturer's specifications?
The researchers found that the mean time of two hundred cars was:
According to this sample statistic, it is not reasonable to assume that the car can perform to the specifications of the manufacturer. This is because the calculated mean run time is far below the desired average quarter mile run time. The time that was calculated in the experiment represents the test statistic, while the manufacturer's desired quarter mile run time represents the population parameter. The statistic represents a single experiment used to test a hypothesis that stated: the new car has an average quarter mile run time of
This hypothesis was tested by a single experiment that measured the quarter mile run time of two hundred stock cars. Replication of this experiment would gather means of multiple samples. If the researchers were able to gather a census of the quarter mile run time of the population, then they could create a standard curve:
A standard distribution represents a distribution of the means; furthermore, its mean (i.e. the mean of the means) is hypothetically equal to the population parameter mean.
It is costly and unlikely that a researcher will be able to take a census of all the instances of a particular phenomenon. As a result, many researchers will take a limited sample and fit a standard curve to it in order to gather a representative estimate of a populations mean—in this case quarter mile run times. In this example, researchers could gather the mean of one hundred experiments and create the following graph:
We can see in this graph that the sample statistic doesn't hover around the center—mean of the means—of the graph; therefore, it is not reasonable to assume that this statistic supports the hypothesis that stated that the car could meet designer specifications.
Example Question #2 : Estimate Population Mean And Margin Of Error: Ccss.Math.Content.Hss Ic.B.4
A car manufacturer wants to produce a sports car that has an average quarter mile run time of:
Researchers decide to randomly sample two hundred cars off of the stock production line. They find that these cars' mean quarter mile run time is as follows:
Is it reasonable to say that the car performs to the manufacturer's specifications?
No
Cannot be determined
Yes
No
In order to solve this question, we need to learn how to infer population parameters from sample statistics. We will do this by reviewing the definitions of parameters and statistics, recalling the processes associated with the scientific method, and by interrogating the information in the problem.
First, let's discuss what is meant by the term population. In statistics, a "population" is described as the entire group that is to be studied. An example of a population in the natural sciences would be every giant panda of the species Ailuropoda melanoleuca in the wild (1864 individuals according to the World Wildlife Foundation)—not captivity. Now, let's identify what is meant by the term population parameter. A "population parameter" is a statistic that is found by sampling the entire population. For example, the mean weight of the entire wild population of giant pandas in the world would be an example of a population parameter (i.e. the mean weight of all 1864 pandas). Next, we will discuss sample populations and statistics.
A "sample" is the subset of a population that is being studied. For example, researchers for a university want to study giant pandas in the wild but can only access a group of 100 pandas sampled in Sichuan, China. Data collected from this particular study would be known as a sample statistic (e.g. the mean weight of pandas in the Sichuan region). It is important to note that the external validity of some sample statistics are hindered. The external validity of a statistic is its ability to be applied to other samples and remain valid. If locals fed pandas in the Sichuan region, then their mean weight may be greater than those of the southern or northern regions. In this instance, the mean would not be representative of other populations of giant pandas.
Last, we should note that certain sample populations are better than others at predicting population parameters. A population parameter can be considered to be the true statistic of a given population while a sample statistic is only an estimate of a part or subset of the population. Simple random samples are good predictors of population parameters and can be used to estimate them. They are collected when every member in a population has an equal chance of being chosen (e.g. randomly selecting 100 of the 1864 pandas in the world).
Next, we shall observe the steps associated with the scientific method.
In this method, researchers observe a phenomenon and develop a tentative explanation for it. Afterwards, they develop an experiment, which assigns subjects or variables to control and treatment groups. Experiments measure the change that an independent variable (i.e. treatment) has on a dependent variable (i.e. subject or phenomena under investigation). Scientists repeat or replicate these experiments multiple times in order gather data for statistical analysis. Scientists analyze the data and then use this evidence to either support or refute a hypothesis. Hypotheses can only be supported or refuted: never proven. This is because scientific investigation can only gather evidence for or against a particular phenomenon. Even theories such as gravity and natural selection have not been proven—they are simply supported by an almost endless amount of scientific evidence.
Last, let's use this information to solve the following problem:
A car manufacturer wants to produce a sports car that has the following average quarter mile run time:
Researchers decide to randomly sample two hundred cars off of the stock production line. They find that these cars' quarter mile run mean time is as follows:
Is it reasonable to say that the car performs to the manufacturer's specifications?
The researchers found that the mean time of two hundred cars was:
According to this sample statistic, it is not reasonable to assume that the car can perform to the specifications of the manufacturer. This is because the calculated mean run time is far above the desired average quarter mile run time. The time that was calculated in the experiment represents the test statistic, while the manufacturer's desired quarter mile run time represents the population parameter. The statistic represents a single experiment used to test a hypothesis that stated: the new car has an average quarter mile run time of
This hypothesis was tested by a single experiment that measured the quarter mile run time of two hundred stock cars. Replication of this experiment would gather means of multiple samples. If the researchers were able to gather a census of the quarter mile run time of the population, then they could create a standard curve:
A standard distribution represents a distribution of the means; furthermore, its mean (i.e. the mean of the means) is hypothetically equal to the population parameter mean.
It is costly and unlikely that a researcher will be able to take a census of all the instances of a particular phenomenon. As a result, many researchers will take a limited sample and fit a standard curve to it in order to gather a representative estimate of a populations mean—in this case quarter mile run times. In this example, researchers could gather the mean of one hundred experiments and create the following graph:
We can see in this graph that the sample statistic doesn't hover around the center—mean of the means—of the graph; therefore, it is not reasonable to assume that this statistic supports the hypothesis that stated that the car could meet designer specifications.
Example Question #6 : Estimate Population Mean And Margin Of Error: Ccss.Math.Content.Hss Ic.B.4
A car manufacturer wants to produce a sports car that has an average quarter mile run time of:
Researchers decide to randomly sample two hundred cars off of the stock production line. They find that these cars' mean quarter mile run time is as follows:
Is it reasonable to say that the car performs to the manufacturer's specifications?
Yes
No
Cannot be determined
No
In order to solve this question, we need to learn how to infer population parameters from sample statistics. We will do this by reviewing the definitions of parameters and statistics, recalling the processes associated with the scientific method, and by interrogating the information in the problem.
First, let's discuss what is meant by the term population. In statistics, a "population" is described as the entire group that is to be studied. An example of a population in the natural sciences would be every giant panda of the species Ailuropoda melanoleuca in the wild (1864 individuals according to the World Wildlife Foundation)—not captivity. Now, let's identify what is meant by the term population parameter. A "population parameter" is a statistic that is found by sampling the entire population. For example, the mean weight of the entire wild population of giant pandas in the world would be an example of a population parameter (i.e. the mean weight of all 1864 pandas). Next, we will discuss sample populations and statistics.
A "sample" is the subset of a population that is being studied. For example, researchers for a university want to study giant pandas in the wild but can only access a group of 100 pandas sampled in Sichuan, China. Data collected from this particular study would be known as a sample statistic (e.g. the mean weight of pandas in the Sichuan region). It is important to note that the external validity of some sample statistics are hindered. The external validity of a statistic is its ability to be applied to other samples and remain valid. If locals fed pandas in the Sichuan region, then their mean weight may be greater than those of the southern or northern regions. In this instance, the mean would not be representative of other populations of giant pandas.
Last, we should note that certain sample populations are better than others at predicting population parameters. A population parameter can be considered to be the true statistic of a given population while a sample statistic is only an estimate of a part or subset of the population. Simple random samples are good predictors of population parameters and can be used to estimate them. They are collected when every member in a population has an equal chance of being chosen (e.g. randomly selecting 100 of the 1864 pandas in the world).
Next, we shall observe the steps associated with the scientific method.
In this method, researchers observe a phenomenon and develop a tentative explanation for it. Afterwards, they develop an experiment, which assigns subjects or variables to control and treatment groups. Experiments measure the change that an independent variable (i.e. treatment) has on a dependent variable (i.e. subject or phenomena under investigation). Scientists repeat or replicate these experiments multiple times in order gather data for statistical analysis. Scientists analyze the data and then use this evidence to either support or refute a hypothesis. Hypotheses can only be supported or refuted: never proven. This is because scientific investigation can only gather evidence for or against a particular phenomenon. Even theories such as gravity and natural selection have not been proven—they are simply supported by an almost endless amount of scientific evidence.
Last, let's use this information to solve the following problem:
A car manufacturer wants to produce a sports car that has the following average quarter mile run time:
Researchers decide to randomly sample two hundred cars off of the stock production line. They find that these cars' quarter mile run mean time is as follows:
Is it reasonable to say that the car performs to the manufacturer's specifications?
The researchers found that the mean time of two hundred cars was:
According to this sample statistic, it is not reasonable to assume that the car can perform to the specifications of the manufacturer. This is because the calculated mean run time is far above the desired average quarter mile run time. The time that was calculated in the experiment represents the test statistic, while the manufacturer's desired quarter mile run time represents the population parameter. The statistic represents a single experiment used to test a hypothesis that stated: the new car has an average quarter mile run time of
This hypothesis was tested by a single experiment that measured the quarter mile run time of two hundred stock cars. Replication of this experiment would gather means of multiple samples. If the researchers were able to gather a census of the quarter mile run time of the population, then they could create a standard curve:
A standard distribution represents a distribution of the means; furthermore, its mean (i.e. the mean of the means) is hypothetically equal to the population parameter mean.
It is costly and unlikely that a researcher will be able to take a census of all the instances of a particular phenomenon. As a result, many researchers will take a limited sample and fit a standard curve to it in order to gather a representative estimate of a populations mean—in this case quarter mile run times. In this example, researchers could gather the mean of one hundred experiments and create the following graph:
We can see in this graph that the sample statistic doesn't hover around the center—mean of the means—of the graph; therefore, it is not reasonable to assume that this statistic supports the hypothesis that stated that the car could meet designer specifications.
Example Question #7 : Estimate Population Mean And Margin Of Error: Ccss.Math.Content.Hss Ic.B.4
A car manufacturer wants to produce a sports car that has an average quarter mile run time of:
Researchers decide to randomly sample two hundred cars off of the stock production line. They find that these cars' mean quarter mile run time is as follows:
Is it reasonable to say that the car performs to the manufacturer's specifications?
No
Cannot be determined
Yes
Yes
In order to solve this question, we need to learn how to infer population parameters from sample statistics. We will do this by reviewing the definitions of parameters and statistics, recalling the processes associated with the scientific method, and by interrogating the information in the problem.
First, let's discuss what is meant by the term population. In statistics, a "population" is described as the entire group that is to be studied. An example of a population in the natural sciences would be every giant panda of the species Ailuropoda melanoleuca in the wild (1864 individuals according to the World Wildlife Foundation)—not captivity. Now, let's identify what is meant by the term population parameter. A "population parameter" is a statistic that is found by sampling the entire population. For example, the mean weight of the entire wild population of giant pandas in the world would be an example of a population parameter (i.e. the mean weight of all 1864 pandas). Next, we will discuss sample populations and statistics.
A "sample" is the subset of a population that is being studied. For example, researchers for a university want to study giant pandas in the wild but can only access a group of 100 pandas sampled in Sichuan, China. Data collected from this particular study would be known as a sample statistic (e.g. the mean weight of pandas in the Sichuan region). It is important to note that the external validity of some sample statistics are hindered. The external validity of a statistic is its ability to be applied to other samples and remain valid. If locals fed pandas in the Sichuan region, then their mean weight may be greater than those of the southern or northern regions. In this instance, the mean would not be representative of other populations of giant pandas.
Last, we should note that certain sample populations are better than others at predicting population parameters. A population parameter can be considered to be the true statistic of a given population while a sample statistic is only an estimate of a part or subset of the population. Simple random samples are good predictors of population parameters and can be used to estimate them. They are collected when every member in a population has an equal chance of being chosen (e.g. randomly selecting 100 of the 1864 pandas in the world).
Next, we shall observe the steps associated with the scientific method.
In this method, researchers observe a phenomenon and develop a tentative explanation for it. Afterwards, they develop an experiment, which assigns subjects or variables to control and treatment groups. Experiments measure the change that an independent variable (i.e. treatment) has on a dependent variable (i.e. subject or phenomena under investigation). Scientists repeat or replicate these experiments multiple times in order gather data for statistical analysis. Scientists analyze the data and then use this evidence to either support or refute a hypothesis. Hypotheses can only be supported or refuted: never proven. This is because scientific investigation can only gather evidence for or against a particular phenomenon. Even theories such as gravity and natural selection have not been proven—they are simply supported by an almost endless amount of scientific evidence.
Last, let's use this information to solve the following problem:
A car manufacturer wants to produce a sports car that has the following average quarter mile run time:
Researchers decide to randomly sample two hundred cars off of the stock production line. They find that these cars' quarter mile run mean time is as follows:
Is it reasonable to say that the car performs to the manufacturer's specifications?
The researchers found that the mean time of two hundred cars was:
According to this sample statistic, it is reasonable to assume that the car can and will perform to the specifications of the manufacturer. This is because the calculated mean run time is only slightly above the desired average quarter mile run time. The time that was calculated in the experiment represents the test statistic, while the manufacturer's desired quarter mile run time represents the population parameter. The statistic represents a single experiment used to test a hypothesis that stated: the new car has an average quarter mile run time of
This hypothesis was tested by a single experiment that measured the quarter mile run time of two hundred stock cars. Replication of this experiment would gather means of multiple samples. If the researchers were able to gather a census of the quarter mile run time of the population, then they could create a standard curve:
A standard distribution represents a distribution of the means; furthermore, its mean (i.e. the mean of the means) is hypothetically equal to the population parameter mean.
It is costly and unlikely that a researcher will be able to take a census of all the instances of a particular phenomenon. As a result, many researchers will take a limited sample and fit a standard curve to it in order to gather a representative estimate of a populations mean—in this case quarter mile run times. In this example, researchers could gather the mean of one hundred experiments and create the following graph:
We can see in this graph that the sample statistic hovers around the center—mean of the means—of the graph; therefore, it is reasonable to assume that this statistic supports the hypothesis that stated that the car could meet designer specifications.
Example Question #8 : Estimate Population Mean And Margin Of Error: Ccss.Math.Content.Hss Ic.B.4
A car manufacturer wants to produce a sports car that has an average quarter mile run time of:
Researchers decide to randomly sample two hundred cars off of the stock production line. They find that these cars' mean quarter mile run time is as follows:
Is it reasonable to say that the car performs to the manufacturer's specifications?
Yes
No
Cannot be determined
Yes
In order to solve this question, we need to learn how to infer population parameters from sample statistics. We will do this by reviewing the definitions of parameters and statistics, recalling the processes associated with the scientific method, and by interrogating the information in the problem.
First, let's discuss what is meant by the term population. In statistics, a "population" is described as the entire group that is to be studied. An example of a population in the natural sciences would be every giant panda of the species Ailuropoda melanoleuca in the wild (1864 individuals according to the World Wildlife Foundation)—not captivity. Now, let's identify what is meant by the term population parameter. A "population parameter" is a statistic that is found by sampling the entire population. For example, the mean weight of the entire wild population of giant pandas in the world would be an example of a population parameter (i.e. the mean weight of all 1864 pandas). Next, we will discuss sample populations and statistics.
A "sample" is the subset of a population that is being studied. For example, researchers for a university want to study giant pandas in the wild but can only access a group of 100 pandas sampled in Sichuan, China. Data collected from this particular study would be known as a sample statistic (e.g. the mean weight of pandas in the Sichuan region). It is important to note that the external validity of some sample statistics are hindered. The external validity of a statistic is its ability to be applied to other samples and remain valid. If locals fed pandas in the Sichuan region, then their mean weight may be greater than those of the southern or northern regions. In this instance, the mean would not be representative of other populations of giant pandas.
Last, we should note that certain sample populations are better than others at predicting population parameters. A population parameter can be considered to be the true statistic of a given population while a sample statistic is only an estimate of a part or subset of the population. Simple random samples are good predictors of population parameters and can be used to estimate them. They are collected when every member in a population has an equal chance of being chosen (e.g. randomly selecting 100 of the 1864 pandas in the world).
Next, we shall observe the steps associated with the scientific method.
In this method, researchers observe a phenomenon and develop a tentative explanation for it. Afterwards, they develop an experiment, which assigns subjects or variables to control and treatment groups. Experiments measure the change that an independent variable (i.e. treatment) has on a dependent variable (i.e. subject or phenomena under investigation). Scientists repeat or replicate these experiments multiple times in order gather data for statistical analysis. Scientists analyze the data and then use this evidence to either support or refute a hypothesis. Hypotheses can only be supported or refuted: never proven. This is because scientific investigation can only gather evidence for or against a particular phenomenon. Even theories such as gravity and natural selection have not been proven—they are simply supported by an almost endless amount of scientific evidence.
Last, let's use this information to solve the following problem:
A car manufacturer wants to produce a sports car that has the following average quarter mile run time:
Researchers decide to randomly sample two hundred cars off of the stock production line. They find that these cars' quarter mile run mean time is as follows:
Is it reasonable to say that the car performs to the manufacturer's specifications?
The researchers found that the mean time of two hundred cars was:
According to this sample statistic, it is reasonable to assume that the car can and will perform to the specifications of the manufacturer. This is because the calculated mean run time is only slightly below the desired average quarter mile run time. The time that was calculated in the experiment represents the test statistic, while the manufacturer's desired quarter mile run time represents the population parameter. The statistic represents a single experiment used to test a hypothesis that stated: the new car has an average quarter mile run time of
This hypothesis was tested by a single experiment that measured the quarter mile run time of two hundred stock cars. Replication of this experiment would gather means of multiple samples. If the researchers were able to gather a census of the quarter mile run time of the population, then they could create a standard curve:
A standard distribution represents a distribution of the means; furthermore, its mean (i.e. the mean of the means) is hypothetically equal to the population parameter mean.
It is costly and unlikely that a researcher will be able to take a census of all the instances of a particular phenomenon. As a result, many researchers will take a limited sample and fit a standard curve to it in order to gather a representative estimate of a populations mean—in this case quarter mile run times. In this example, researchers could gather the mean of one hundred experiments and create the following graph:
We can see in this graph that the sample statistic hovers around the center—mean of the means—of the graph; therefore, it is reasonable to assume that this statistic supports the hypothesis that stated that the car could meet designer specifications.
Example Question #211 : High School: Statistics & Probability
A car manufacturer wants to produce a sports car that has an average quarter mile run time of:
Researchers decide to randomly sample two hundred cars off of the stock production line. They find that these cars' mean quarter mile run time is as follows:
Is it reasonable to say that the car performs to the manufacturer's specifications?
Cannot be determined
No
Yes
Yes
In order to solve this question, we need to learn how to infer population parameters from sample statistics. We will do this by reviewing the definitions of parameters and statistics, recalling the processes associated with the scientific method, and by interrogating the information in the problem.
First, let's discuss what is meant by the term population. In statistics, a "population" is described as the entire group that is to be studied. An example of a population in the natural sciences would be every giant panda of the species Ailuropoda melanoleuca in the wild (1864 individuals according to the World Wildlife Foundation)—not captivity. Now, let's identify what is meant by the term population parameter. A "population parameter" is a statistic that is found by sampling the entire population. For example, the mean weight of the entire wild population of giant pandas in the world would be an example of a population parameter (i.e. the mean weight of all 1864 pandas). Next, we will discuss sample populations and statistics.
A "sample" is the subset of a population that is being studied. For example, researchers for a university want to study giant pandas in the wild but can only access a group of 100 pandas sampled in Sichuan, China. Data collected from this particular study would be known as a sample statistic (e.g. the mean weight of pandas in the Sichuan region). It is important to note that the external validity of some sample statistics are hindered. The external validity of a statistic is its ability to be applied to other samples and remain valid. If locals fed pandas in the Sichuan region, then their mean weight may be greater than those of the southern or northern regions. In this instance, the mean would not be representative of other populations of giant pandas.
Last, we should note that certain sample populations are better than others at predicting population parameters. A population parameter can be considered to be the true statistic of a given population while a sample statistic is only an estimate of a part or subset of the population. Simple random samples are good predictors of population parameters and can be used to estimate them. They are collected when every member in a population has an equal chance of being chosen (e.g. randomly selecting 100 of the 1864 pandas in the world).
Next, we shall observe the steps associated with the scientific method.
In this method, researchers observe a phenomenon and develop a tentative explanation for it. Afterwards, they develop an experiment, which assigns subjects or variables to control and treatment groups. Experiments measure the change that an independent variable (i.e. treatment) has on a dependent variable (i.e. subject or phenomena under investigation). Scientists repeat or replicate these experiments multiple times in order gather data for statistical analysis. Scientists analyze the data and then use this evidence to either support or refute a hypothesis. Hypotheses can only be supported or refuted: never proven. This is because scientific investigation can only gather evidence for or against a particular phenomenon. Even theories such as gravity and natural selection have not been proven—they are simply supported by an almost endless amount of scientific evidence.
Last, let's use this information to solve the following problem:
A car manufacturer wants to produce a sports car that has the following average quarter mile run time:
Researchers decide to randomly sample two hundred cars off of the stock production line. They find that these cars' quarter mile run mean time is as follows:
Is it reasonable to say that the car performs to the manufacturer's specifications?
The researchers found that the mean time of two hundred cars was:
According to this sample statistic, it is reasonable to assume that the car can and will perform to the specifications of the manufacturer. This is because the calculated mean run time is only slightly below the desired average quarter mile run time. The time that was calculated in the experiment represents the test statistic, while the manufacturer's desired quarter mile run time represents the population parameter. The statistic represents a single experiment used to test a hypothesis that stated: the new car has an average quarter mile run time of
This hypothesis was tested by a single experiment that measured the quarter mile run time of two hundred stock cars. Replication of this experiment would gather means of multiple samples. If the researchers were able to gather a census of the quarter mile run time of the population, then they could create a standard curve:
A standard distribution represents a distribution of the means; furthermore, its mean (i.e. the mean of the means) is hypothetically equal to the population parameter mean.
It is costly and unlikely that a researcher will be able to take a census of all the instances of a particular phenomenon. As a result, many researchers will take a limited sample and fit a standard curve to it in order to gather a representative estimate of a populations mean—in this case quarter mile run times. In this example, researchers could gather the mean of one hundred experiments and create the following graph:
We can see in this graph that the sample statistic hovers around the center—mean of the means—of the graph; therefore, it is reasonable to assume that this statistic supports the hypothesis that stated that the car could meet designer specifications.
Example Question #212 : High School: Statistics & Probability
A car manufacturer wants to produce a sports car that has an average quarter mile run time of:
Researchers decide to randomly sample two hundred cars off of the stock production line. They find that these cars' mean quarter mile run time is as follows:
Is it reasonable to say that the car performs to the manufacturer's specifications?
No
Yes
Cannot be determined
No
In order to solve this question, we need to learn how to infer population parameters from sample statistics. We will do this by reviewing the definitions of parameters and statistics, recalling the processes associated with the scientific method, and by interrogating the information in the problem.
First, let's discuss what is meant by the term population. In statistics, a "population" is described as the entire group that is to be studied. An example of a population in the natural sciences would be every giant panda of the species Ailuropoda melanoleuca in the wild (1864 individuals according to the World Wildlife Foundation)—not captivity. Now, let's identify what is meant by the term population parameter. A "population parameter" is a statistic that is found by sampling the entire population. For example, the mean weight of the entire wild population of giant pandas in the world would be an example of a population parameter (i.e. the mean weight of all 1864 pandas). Next, we will discuss sample populations and statistics.
A "sample" is the subset of a population that is being studied. For example, researchers for a university want to study giant pandas in the wild but can only access a group of 100 pandas sampled in Sichuan, China. Data collected from this particular study would be known as a sample statistic (e.g. the mean weight of pandas in the Sichuan region). It is important to note that the external validity of some sample statistics are hindered. The external validity of a statistic is its ability to be applied to other samples and remain valid. If locals fed pandas in the Sichuan region, then their mean weight may be greater than those of the southern or northern regions. In this instance, the mean would not be representative of other populations of giant pandas.
Last, we should note that certain sample populations are better than others at predicting population parameters. A population parameter can be considered to be the true statistic of a given population while a sample statistic is only an estimate of a part or subset of the population. Simple random samples are good predictors of population parameters and can be used to estimate them. They are collected when every member in a population has an equal chance of being chosen (e.g. randomly selecting 100 of the 1864 pandas in the world).
Next, we shall observe the steps associated with the scientific method.
In this method, researchers observe a phenomenon and develop a tentative explanation for it. Afterwards, they develop an experiment, which assigns subjects or variables to control and treatment groups. Experiments measure the change that an independent variable (i.e. treatment) has on a dependent variable (i.e. subject or phenomena under investigation). Scientists repeat or replicate these experiments multiple times in order gather data for statistical analysis. Scientists analyze the data and then use this evidence to either support or refute a hypothesis. Hypotheses can only be supported or refuted: never proven. This is because scientific investigation can only gather evidence for or against a particular phenomenon. Even theories such as gravity and natural selection have not been proven—they are simply supported by an almost endless amount of scientific evidence.
Last, let's use this information to solve the following problem:
A car manufacturer wants to produce a sports car that has the following average quarter mile run time:
Researchers decide to randomly sample two hundred cars off of the stock production line. They find that these cars' quarter mile run mean time is as follows:
Is it reasonable to say that the car performs to the manufacturer's specifications?
The researchers found that the mean time of two hundred cars was:
According to this sample statistic, it is not reasonable to assume that the car can perform to the specifications of the manufacturer. This is because the calculated mean run time is far above the desired average quarter mile run time. The time that was calculated in the experiment represents the test statistic, while the manufacturer's desired quarter mile run time represents the population parameter. The statistic represents a single experiment used to test a hypothesis that stated: the new car has an average quarter mile run time of
This hypothesis was tested by a single experiment that measured the quarter mile run time of two hundred stock cars. Replication of this experiment would gather means of multiple samples. If the researchers were able to gather a census of the quarter mile run time of the population, then they could create a standard curve:
A standard distribution represents a distribution of the means; furthermore, its mean (i.e. the mean of the means) is hypothetically equal to the population parameter mean.
It is costly and unlikely that a researcher will be able to take a census of all the instances of a particular phenomenon. As a result, many researchers will take a limited sample and fit a standard curve to it in order to gather a representative estimate of a populations mean—in this case quarter mile run times. In this example, researchers could gather the mean of one hundred experiments and create the following graph:
We can see in this graph that the sample statistic doesn't hover around the center—mean of the means—of the graph; therefore, it is not reasonable to assume that this statistic supports the hypothesis that stated that the car could meet designer specifications.
Certified Tutor
All Common Core: High School - Statistics and Probability Resources
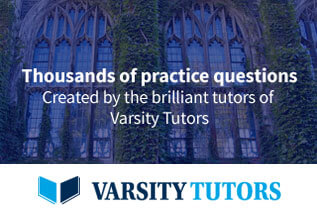